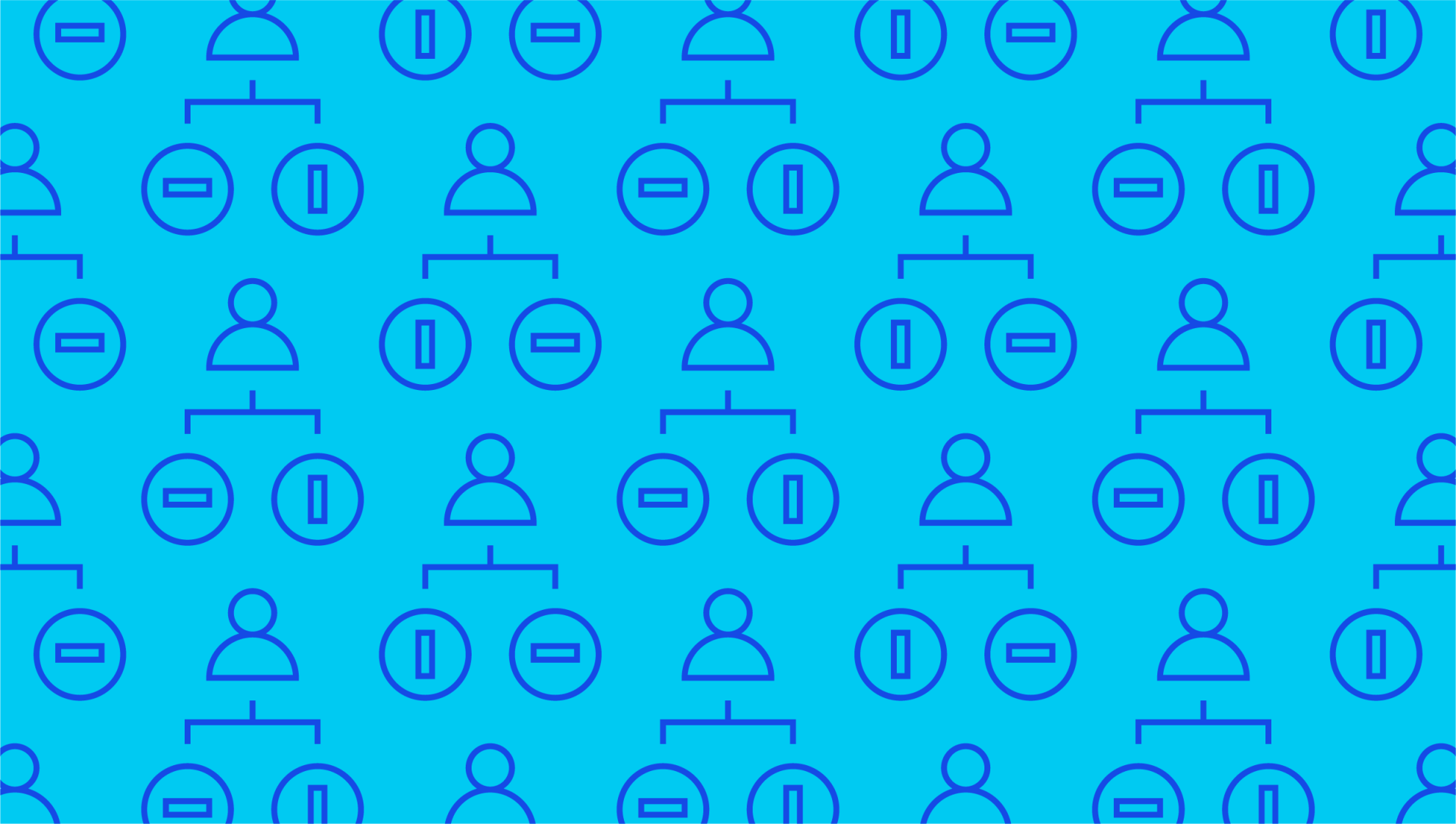
Data cleaning & enrichment: What you need to know
Last editedMar 2023 8 min read
Data cleaning is a procedure that aims to remove imprecise, duplicate or incorrect information from a dataset. This can be achieved through different strategies, with the intent of improving the quality of the data to facilitate decision-making processes that rely on that same data.
We are definitely in the era of big data, and it’s easy to observe how information has become increasingly more important in our daily lives. Data is now more readily accessible than ever, coming in greater variety and volume.
Perhaps it would be safe to say that more data is synonymous with better data. However, that’s not usually the case. Although ideally, FinTechs are more than capable of putting data to good use, it’s not just the quantity that matters. Quality of information plays the most relevant role, as it allows a greater deal of control and consistency.
In big data, the term “veracity” refers to the quality of the data. Information now arises from so many sources, making it very hard to categorise and link. In order to come up with proper innovative products and services, businesses need to find correlations between multiple data streams.
This is not so much about how much data you have, but how you use it. The million-dollar question here is how can FinTechs make sense of all the information they collect? This is where data cleaning, or cleansing, comes in.
In this article, we will try to demonstrate how open banking and data cleaning are tightly related, and how one can help the other to grow and bring the most benefits to customers, businesses, and legacy institutions alike.
What is open banking?
Open bankingenables third-party payment services and financial service providers to access basic consumer banking information, such as transactions and payment history. This process is made possible through the use of application programming interfaces (APIs). Open banking technology has paved the way to a wide range of apps and third-party services that greatly enhance the user experience.
Not only it offers faster, more personalised banking services, but, most importantly, shifts the balance of power to the side of the end-user. Users can decide if and when they want to share their information. By doing so, they are previewed to services that upgrade their day-to-day life, putting them in charge of their financials.
Open banking uses the revised Payment Services Directive (PSD2) as a regulatory framework for electronic payment services. This legislation set the foundation for what we now know as open banking, but that has been merely the beginning. PSD3 is coming and could change everything, from access to account information, fight against payments fraud, and regulate new products and services.
What is data cleaning?
The basics of data cleaning (or data cleansing) are actually quite simple to understand. Imagine you are presented with a complex database made up of unstructured, duplicate or even erroneous information.
There is really not much you can do with that, at least not before you apply some sort of action that makes it consistent, improving the quality of your data. This can be achieved either by eliminating superfluous information or by completing the dataset, filling in the gaps.
Since big data relies on many sources to create datasets, you may need to aggregate the information to have a logical framework to use as a starting point. Data wrangling will also remove errors from datasets, helping to make data more understandable and easy to analyse.
Data cleaning consists of different approaches, both manual and automated, that tend to vary from team to team.The importance of this process is critical to the success of modern businesses for several reasons. Here are some of the most important ones:
Significantly reduces the time and resources of overseeing and filtering processes
Provides businesses with clean data that can be easily visualised
Enhances dynamic decision-making
Increases the quality of information and team productivity
Is data cleaning important?
Whatever strategy you use, there is only one bottom line: to improve data quality, in order to provide more consistent and reliable information that will serve as a baseline for decision-making. Uniformity makes data more actionable, helping people and businesses to make better decisions.
Data cleaning is invaluable to the success of products and services in today’s financial markets, as well as for marketing teams, sales reps, or operational workers.
Taking the necessary steps to cleanse databases can even have a dramatic impact on organisational costs, reducing bad strategies and operational setbacks. Structured data offers the most valuable information to add value to a business or product.
Data-driven businesses should take data cleaning as a foundational step to improve their offerings. With data management taking the front seat when it comes to being one step ahead of the competition, for sure it’s a worthy investment.
Here’s how the data cleaning process works:
Inspection and profiling — gathering the data comes first. There is an initial assessment of the quality and picks out errors, discrepancies and other problems.
Cleaning — this is the meat and potatoes of the entire process. This is where duplicates are erased, irrelevant information is disregarded, and valuable data is grouped together.
Verification — after the cleaning process is completed, what follows is verification of the process that the outcome is indeed what you’re looking for and falls under the right rules and regulations.
Reporting — reporting refers to the process of visualising the findings, giving you insights into what the data were initially and what the result was after the cleaning was done.
At which phase does data cleaning occur?
There is no single answer to this question, as data cleaning can occur at different stages in the open banking process. However, it is generally accepted that data transformation should take place before any analysis or decision-making is conducted.
This ensures that the data used for these purposes is of the highest quality, accuracy, and doesn't have missing values. On the other hand, it may also be conducted after initial analysis has been completed, in order to improve the results of this analysis.
It is important to note that not all data cleaning is created equal, as some banks and service providers do a better job of it than others. When choosing an open banking provider, it is critical to do your research, make sure that you are getting the best data quality possible, and no inconsistent data.
Data cleaning in open banking: you can’t have one without the other
Open banking is probably the main technology behind an extraordinary boost in innovation in the banking and payments markets. One of its main goals is to enable better financial decisions and increase financial literacy.
To do this, open banking heavily relies on the financial data it gathers from banks and other legacy financial institutions. Data science is capable of adding value through the identification of patterns in datasets. Here are some practical examples of how open banking leverages data science:
Enables applications that offer valuable insights about spendings and income;
Enables faster access to credit by facilitating risk assessment and affordability estimations;
Enables advanced KYC (Know Your Customer) and identity verification, boosting security;
Multiple streams of data require an extended effort to identify patterns and inconsistencies through millions and millions of strings of information. Artificial Intelligence and Machine Learning are some of the tools that businesses can use to promote creative ways of adding value to open banking data.
A careful thought-out approach to data cleaning can serve as a starting point to build personalised financial products and services, one of the core values of open banking.
Data ownership and control shifted greatly with open banking, giving back customers the power to choose what data to share, and with whom. Structured data is of capital importance to open banking, as it’s an invaluable tool to help improve people’s lives.
The benefits of data cleaning for open banking
Data cleaning can help open banking protect reputation, minimise compliance risks, and maximise business growth. More than that, it will help with one of the most important pillars of modern business: decision-making.
Real-time decision-making
What is data needed and used for, if you dig down to the bottom of it? To make decisions. Accessing clean, up-to-date, and quality data can trigger a seamless, instant decision-making process that helps businesses stay ahead of the competition.
What does data cleaning mean for your business?
When you are looking at raw data sent over by banks, it doesn't take a long time until you understand it's not possible to work with it. The cleansing process allows your business to become more efficient, faster, and a lot more reliable when analysing transaction information.
With data cleansing, you will be able to make the most of each data point, no matter the volume of information. This is one of the biggest bottlenecks of a raw data set, which presents itself not only barely usable, but also impossible to scale up.
The challenges of data cleaning in open banking
If data cleaning is such a brilliant idea, why is it not widely implemented? The answer is simple: there are a lot of extenuating variables that make automation a challenging task. Data cleansing is considered the most challenging thing about open banking, and here are the main reasons why:
Different metadata for different payment methods. For example, bank transfers, mobile transfers, credit, and debit cards (physical or digital), prepaid cards, and electronic cheques.
Countless payment gateways use their own taxonomies, even for similar services
Highly unstructured bank account transaction information
The banks have different approaches to storing data
Pros and Cons of data cleansing
Pros:
Ensures that data is complete and accurate
Reduces redundancy and inconsistencies
Improves data quality and decision-making
Helps organisations meet compliance requirements
Facilitates data sharing and integration
Cons:
Can be time-consuming and resource-intensive
Requires specialised skills and knowledge
May require significant changes to data management processes
Can be disruptive to business operations
Can create new data quality problems
Data enrichment: what does it mean?
Data enrichment is the process by which supplementary levels of insights are added to raw sets of data. This is particularly useful to complete missing or partial information, and can be achieved by incorporating data from external authoritative sources into an existing database.
One can argue that data enrichment is today perhaps one of the most influential aspects of business, with its impact reaching areas like sales, of course, but also customer service or even marketing.
Why is data enrichment important?
Enriched data is at the core of most decisions within a company, serving as a backbone for sustainable growth and long-term profit strategies. This type of data is easier to observe and categorise, contributing to the overall organisation of your business and the quality of your products and services.
The more structured data you have, the better will your decisions be. Let’s take financial businesses as an example. The data you gather from your customers is registered in its raw form. When customer data is collected and stored, it's pretty much useless.
It's only after passing through data cleaning processesthat this information is enriched, to provide it additional useful knowledge. Data enrichment adds value to raw datasets, helping you get a better understanding of your customers, and, in the particular case of the financial industry, of their financial lives — all without having to ask them for more information.
What are the benefits of data enrichment?
Data enrichment should be seen as a key competitive advantage for those who use this type of resources, as it helps improve crucial business areas:
Know more about your customers, reducing the friction of constantly having to ask them to fill out lengthy forms;
Promote a seamless customer journey, removing obstacles that can lead to abandonment in its various forms;
Improve customer experience, by anticipating their needs and providing them with tailored products and services;
Adequate data enrichment tools deliver results in real-time, saving time and money for all those involved;
Store only what you need, saving the information that has some kind of value to your organisation;
Boost sales, by having an updated and relevant contact list always at your disposal, increasing efficiency;
Improve marketing efforts, by relying on targeted marketing that directly resonates with your ideal customer profile;
Data Enrichment in open banking
When we talk about data enrichment in the context of open banking, we are basically referring to a process by which raw financial data is transformed into actionable information. This is done by cleaning transactional data and categorising it.
Raw transactional data is very hard to work with, mainly because of the abundance of duplicate entries and long strings of text that offer little to no value at first glance. Data enrichment offers a fresh and intelligible new-look over that information, removing the noise, so anyone can understand what’s happening.
After the data has been cleansed, more value can be added to it by attributing categories. It can be advantageous to categorise transaction data because categories allow us to see the bigger picture.
For example, the dinner you had last night at Burger King will be categorised in the “Food and Drinks” category, but your shopping spree at Zara will be added to the “General Merchandise” category. This is invaluable if you wish to deeply understand someone’s personal finances, allowing you to identify patterns and spending habits.
Lending and data enrichment
The lending industry is consistently showing signs of growth, with many businesses looking at these services as a primary way to improve their offerings, get ahead of the competition, and generally take their projects to the next level.
One of the most important aspects of lending is credit scoring, which is heavily dependent on data enrichment. Lenders often access third-party databases to extrapolate a financial profile that allows them to reduce risk, rejecting those deemed as less likely to pay.
With the help of Account Information Services (AIS), lenders can finally base their decisions on something more than just unstructured databases, and are able to do it in real-time.
Personal Finance Management (PFM) and data enrichment
By categorising transaction data, you can know a lot about one’s spending habits. Enriched information can then be used to come up with better insights that can forever change the way you look at your personal financial management.
These kinds of apps are particularly useful when it comes to educating end-users about goal setting, budgeting, and perhaps most critical, how to make the most out of their money.
Data enrichment products take raw transactional data and deliver clear and intelligible information that can be used to change people’s lives and improve their financial literacy. This is invaluable in today’s macroeconomic scenario.
Concepts like Artificial Intelligence, Machine Learning, prediction or aggregation are used in data enrichment to figure out what data is most relevant and make it actionable.