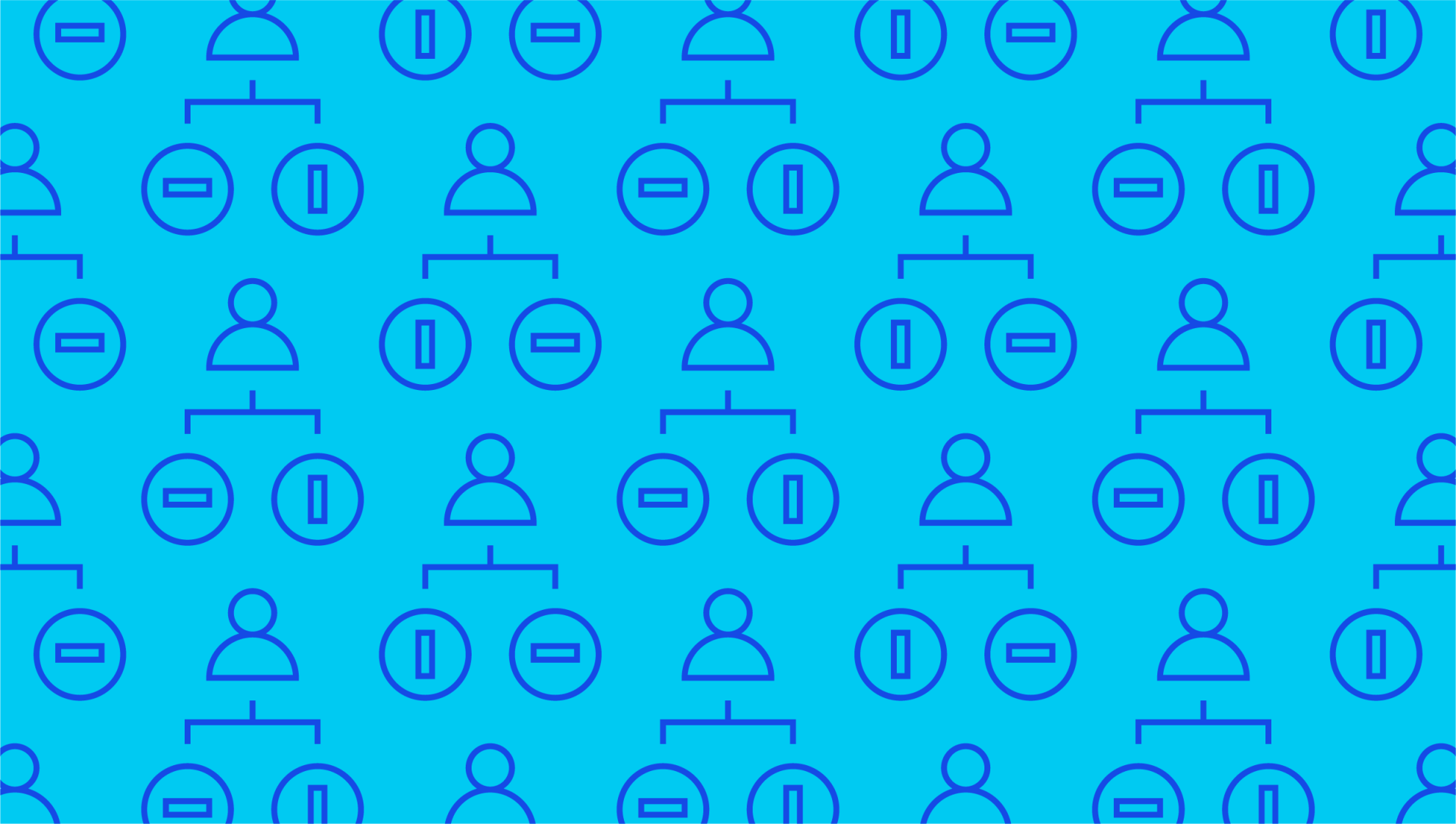
Why are data analysis methods important for your business?
Last editedFeb 2021
Is your business driven by the data? Technology enables us to access a richly detailed treasure trove of information, but do you know what to do with it? Data analysis helps sift through the clutter to extract insights that can help your business grow. Here’s what you need to know about the latest data analysis techniques.
What is data analysis?
Data analysis refers to the process of taking raw data and transforming it into insightful conclusions. Using data analysis methods, businesses can collect, arrange, and analyse data. Scrubbed up and organised, this data yields fresh insights that can prove especially useful when it comes to making business decisions.
The science behind the process of data management is called data analytics. It involves the data analysis itself as well as the tools used to communicate results.
Why is data analysis important?
Data comes from a range of sources, whether it’s market research or customer reviews. The problem with there being such a variety of sources, particularly online, is that these yield mountains of information – not all of it useful. Through data analysis, businesses can sift through the raw information and pick out the most useful components. These are then used to make more efficient business decisions, driven by real-world insight.
Without the data to back them up, business decisions that don’t have a strong foundation carry higher levels of risk. Customer data offers detailed insights about client needs so that you can create more useful products and fine tune your services.
For example, a product development team might carry out a data analysis of customer feedback. This shows that customers interact with products in a certain way, highlighting frustrations and a wish list of new features. The development team uses these insights to implement user-friendly new features, based on evidence rather than intuition.
Data analysis techniques
Just as there are plentiful sources of raw data, there are numerous data analysis techniques. Here are a few of the main analysis methods to try.
Descriptive analysis: This type of analysis looks at historical data to try and work out what happened. For example, a business might track key performance indicators (KPIs) like sales leads or net profit.
Diagnostic analysis: With these types of data analysis methods, you can find out the reasoning behind an event. A diagnostic analysis builds on the descriptive data analysis to uncover the ‘why’ behind the ‘what’. For example, a business might see that leads decreased over the past quarter. A diagnostic analysis would try and determine what went wrong with the marketing strategy.
Predictive analysis: While the previous two methods look at what happened in the past, a predictive analysis tries to speculate about the future. Analysts use past data to form predictions about future trends. For example, you could examine trends in sales figures during the holiday season to make a prediction for this year’s revenue.
Text analysis: Also called data mining, text analysis is an automated form of processing that organises unstructured text data. It sorts text by topic and can extract common keywords. If you have a high volume of customer feedback, text analysis picks out the commonalities to identify trends.
Statistical data analysis: Not all conclusions are relevant. With a statistical data analysis, you can determine if the results are statistically significant or not. To be significant in statistics, an anomaly or result must be verifiable, rather than created by chance.
Prescriptive analysis: This type of data analysis is the most advanced technique because it looks at all of the information available to form a full plan of action. Artificial intelligence is one example of prescriptive data analysis, breaking down high volumes of data and teaching itself how to use this information to make future decisions.
Making the most of a data analysis report
As you can see, there are many different approaches to data analysis. To make the most out of this process, you should begin with clear objectives. This helps refine the type of data you’re collecting and select the best technique to use.
Cleaning your data makes it easier to analyse. This means eliminating things like extra characters, HTML tags, and punctuation marks. Look for data analysis methods that can easily be integrated into your business systems. These will clean the data for you before beginning the analysis.
Finally, you can compile the results into a visual-rich data analysis report. A dashboard provides a handy location for data aggregation, making it easy to view trends at a glance.
We can help
GoCardless helps you automate payment collection, cutting down on the amount of admin your team needs to deal with when chasing invoices. Find out how GoCardless can help you with ad hoc payments or recurring payments.